Early risk analysis saves time and money by avoiding costly mistakes at later implementation and rollout phases of projects. It is important to fully assess the profitability and viability of projects using risk modeling techniques before embarking on them.
Assessing the Risk of Cleantech Projects
Randy Heffernan | Palisade Corporation
What is risk analysis? How does it work?
Risk analysis is a technique that enables companies to judge which risks to take and which ones to avoid, allowing for the best decision making under uncertainty. Using risk analysis, organizations can answer questions like, “What is the probability of profit exceeding $10 million?” or “What are the chances of losing money on this venture?”
Organizations are taking a more strategic approach to risk. Increasingly, companies are adopting more sophisticated techniques to specifically account for, rather than ignore, the inherent uncertainty and unpredictability that characterize risk.
Techniques such as quantitative risk management (QRM) and decision-making under uncertainty (DMU) are widely used to predict uncertain outcomes. Utilizing QRM and DMU requires thinking more quantitatively, with numbers and probabilities, recognizing the reality that uncertainty exists in nearly every decision and accounting for those uncertainties in a quantitative way. Quantitative Risk Analysis (QRA) is becoming an increasingly important tool in planning for risk.
One helpful example of QRA is the use of Monte Carlo simulation. An analytical technique that’s been around since World War II, Monte Carlo simulation is a computational method which, in simple terms, looks at all possible outcomes and identifies the probabilities of different outcomes occurring. Monte Carlo simulation in particular is being applied by a wide range of private companies and government agencies to formulate mitigation strategies.
Monte Carlo simulation enables decision-makers to use ranges of values instead of single-point estimates to represent uncertain values. These ranges are known as probability distributions, and include commonly-used ones like the normal, or “bell curve,” and the uniform, which means that every value in the range has an equal chance of occurring. Depending on the distribution, certain parts of each range are more likely to happen than others.
Monte Carlo simulation also allows for identifying the drivers of risk and ranking them from most to least important based on the impact each has on the bottom-line. This allows decision makers to put resources into mitigating the most important risks instead of wasting time by guessing.
How can companies in the energy industry use risk analysis?
Organizations are investing huge resources to locate and exploit alternate sources of energy. While sustainable energy sectors such as biofuel, solar and wind power can play a crucial gap in bridging the demand and supply for energy, they also have practical challenges. In particular, profit margins are uncertain and tight, and therefore require detailed analysis of complex business cases. The frequent involvement of untested technologies, government incentives and complex, novel supply chains make the outcomes even more unpredictable.
To deal with these challenges, sustainable energy stakeholders must apply probabilistic risk analysis techniques. Commonly applied in petroleum exploration and engineering initiatives, quantitative risk assessment methods can also play a key role in developing profitable business cases for sustainable energy projects. Please see the link below for more information how energy companies are using risk analysis for different purposes:
http://www.palisade.com/cases/blade.asp?caseNav=byIndustry
Is there a minimum project budget that you would consider to begin to benefit from risk analysis?
There is no single number for beginning and benefiting from risk analysis. In fact, one of the core principles of good risk analysis is the avoidance of relying on a single number. The world is in ranges, and so we must think in ranges. The proper budget for a risk analysis initiative would need to take into account the size of the organization, the scope of the risks being analyzed, constraints on available funds, and, of course, the inherent uncertainties. Thus, the ideal budget would take into account a range of values, with confidence intervals, dependent on the organization and its goals.
What are some of the benefits of analyzing risk early on?
Early risk analysis saves time and money by avoiding costly mistakes at later implementation and rollout phases of projects. It is important to fully assess the profitability and viability of projects using risk modeling techniques before embarking on them. Frequently, companies feel pressured to beat the competitors to market, especially in the energy industry, and often skip important risk assessment steps in the planning stages. For the benefit of all stakeholders -- investors, consumers, and the environment – it is absolutely critical to gather experts, historical data and other sources to identify risks worth analyzing.
At what stage of a project do you recommend starting the risk analysis, who should be involved and where does the data come from?
Risk analysis should be included from the very first stages of any project. Without a clear, quantitative understanding of the risks involved, it is impossible to make a go/no-go decision, or even determine whether it’s worth further investment in planning and research. However, risk analysis, like other functions in project management. It is an iterative process, especially for large projects. Just determining the feasibility of a project costs time and money – in man-hours, research, tests, etc. Risk can be determines as best as it can with what is known at the first stage and then those risks can be simulated and analyzed to inform next steps. As a result, organizations can gain new information to feed into the next stage of analysis.
What are the costs involved with risk analysis?
Risk analysis does not need to be capital intensive. Probably, one of the biggest barriers to successful adoption of risk analysis techniques is a cultural one. It’s changing the way people think about risk and about project management in general. Psychologically, and organizationally, most people compartmentalize risk into a siloed function to be done “later.” Howver, risk analysis can only be beneficial with an integrated approach.. Direct costs may involve software procurement and model development, but compared with the projects being analyzed, these expenses are generally negligible. The real challenge is adopting a change in thinking, and that works best when it comes from the top down.
Can you share some examples to illustrate how risk analysis can be an important tool for analyzing the success of clean energy projects?
Sark7, an international consultancy that specializes in integrated analysis for sustainable energy projects is a great example. The consultancy uses risk analysis to bridge the gap between populist enthusiasm surrounding ‘green’ initiatives and the intensive financial risk analysis demanded by capital investors and participants.
A key aspect of Sark7’s value proposition is the in-depth decision-making insight provided by intensive, integrated computer-based modelling and simulation. Sark 7 used risk analysis software to assess the profit potential of sustainability initiatives, separating ‘over-optimistic hype’ from realistic profit margins. Please see the link below for an-indepth case study:
http://www.palisade.com/cases/Sark7.asp
Please explain how Futuremetrics is using risk analysis.
Future Metrics is one of the leading domestic experts in the economics of the production and use of renewable bioenergy. They, along with their partner Innovative Natural Resource Solutions, formed a new company, FutureEnergy Partners, to focus on the economics and risk of wood pellet production. Using risk analysis software, they performed risk analysis simulations to address some very critical concerns regarding the bright future of wood-pellet production:
- How much wood is required to operate a wood-powered plant and produce the appropriate amount of wood pellets to keep up with demand?
- How do they hedge their prices against global wood prices in a fashion that will allow them to turn a profit?
In a very informative case study, FutureMetrics shared its use of Monte Carlo simulation to answer both of those questions and secure a bright (and warm) future for U.S. wood pellet producers.
FutureMetrics Uses @RISK to Hedge Wood Prices in Production of Burning Wood Pellets
Wood pellets are commonly used in Europe to fuel hundreds of thousands of home heating systems. They are also in high demand in Europe in power plants as a carbon neutral substitution for coal. They are comprised of highly densified wood in terms of both volume and energy content in a uniform shape. One of the key input drivers of ROI for a biomass energy project or pellet project is the price of wood. FutureMetrics, a consultancy for project development and policy research in the biomass thermal energy sector, uses Palisade’s @RISK to accurately estimate wood costs. The consultancy uses @RISK to analyze the uncertainty surrounding wood prices and thereby measures the potential excess or shortfall of cash flows in present value terms for upcoming projects.
About FutureMetrics
Based in Bethel, Maine, FutureMetrics is recognized as one of the leading domestic experts in the economics of the production and use of renewable bioenergy. FutureMetrics is also recognized for its expertise in quantifying the financial feasibility of renewable energy projects and quantifying the economic impacts, such as jobs, growth and tax revenues of those projects.
Wood Pellet Popularity and Potential ROI
Recently, FutureMetrics and its partner Innovative Natural Resource Solutions (INRS) formed a new company, FutureEnergy Partners, to focus on the economics and risk of wood pellet production. In the next 10 years, the popularity in burning wood pellets as a heating source is expected to rise. But the significant expected growth in wood pellet demand is for the European electricity generation markets where carbon emission policies penalize coal combustion but not carbon neutral wood pellet fuel. Today, the global consumption of wood pellets is approximately 15 million tons per year. It is expected to exceed 45 million tons by 2020. The largest market for wood pellets exists in Europe, where regulations encourage the use of carbon neutral fuels, like biomass. With such a dramatic jump in global wood pellet consumption predicted in the future, FutureMetrics and INRS, via their partnership in FutureEnergy Partners developed a wood price hedging product that uses @RISK to measure its effectiveness. The model was based on a pellet manufacturing facility, which uses a biomass-fueled combined heat and power plant to power the manufacturing process. To power the facility and produce 300,000 tons of pellets annually, 750,000 tons of wood per year was required.
“I have been using @RISK since the early 1990’s, so I have a perspective on the commitment to the users that the Palisade team has,” said Dr. William Strauss, president and founder of FutureMetrics. “Every new version has new and very useful features that often are based on feedback from users. Palisade’s @RISK software has the richest feature set and has the most relevant tools for analyzing models that contain uncertainty.”
@RISK Offers Insight to Appropriate Price-Hedging
For wood-to-energy or wood pellet manufacturing projects, wood costs are the most important driver of changes in the bottom line. As prices are driven by a variety of factors, FutureMetrics and INRS researched wood price movements and created an econometric model that explained those movements. The parameters that drove the model were all commonly traded futures products, which allowed the companies to develop a strategy to hedge wood price fluctuations. Given the inherent variability in the price hedging model’s inputs, @RISK was utilized to create a comprehensive cash flow model for a wood pellet export project.
In this analysis, the inputs came from two sources:
- The explanatory variables of the wood price equation. The historical time series of wood costs were utilized to determine the proper probability distribution for each series, using @RISK’s distribution fitting feature.
- The actual wood cost time series was also considered, and a probability distribution was also estimated for the price of wood using @RISK. A beta general distribution was used to model the price of wood. That series then drives the cash flow model going out 10 years. The hedged outcome is then compared to the un-hedged outcome.
The project’s success was measured by net present value and return on investment. @RISK enabled the team to see the range of possible values for these metrics in the form of probability distributions, so the actual likelihood of a project making money or losing money could be easily quantified. The @RISK tornado charts assisted FutureMetrics and INRS to quantify the sensitivity of the project to changes in input costs.
Findings Using @RISK
Utilizing @RISK, FutureMetrics and INRS found that a pellet manufacturing plant generating 300,000 tons of pellets per year could expect a median ROI on the all-in capital costs of 25.14 percent over the 20 year life of the project. The companies were also interested in learning the probability that the project would lose money during that same time and in turn, how much could the plant expect to lose?
The research showed that there was a nine percent chance that the plant would have a negative NPV over a the life of the project period.
Figure 1: Without hedging, there was a nine percent probability of loss over a three-year period.
Looking at the sensitivity of the model to wood prices in @RISK, it became apparent that the price of the wood going into the pellet mill was critical. @RISK’s tornado chart on the model showed that for each $8.80 (which equals one standard deviation in the price of the wood) increase in wood prices, the NPV was lowered by $22.96 million. Since the expected NPV was only $35.7 million to begin with, the project was exposed to significant price risk.
Figure 2: @RISK’s tornado graph shows that the price of wood going into the pellet mill had by far the greatest impact on NPV.
This risk can be mitigated by hedging their input wood prices. FutureMetrics and INRS then considered the case of the wood aggregator who buys wood from various sources and resells it to pellet manufacturers at a fixed price. In order for the aggregator to reduce its risks if it is going to offer a stable price to the pellet manufacturers, it too must find a way to hedge against market price fluctuations.
Using an econometric model, a simulated set of wood prices, and @RISK, FutureMetrics and INRS determined that the aggregator had a huge 89.5% change of losing money without a hedge, with a mean expected loss of $143.9 million. With a price hedging strategy in place, the mean loss was drastically reduced to $18,000.
Figure 3: The probability of the aggregator losing money without a hedging strategy to insulate against market price fluctuations is 89%, with a mean loss of $143.9 million.
Figure 4: The wood aggregator’s potential loss with a hedging strategy are greatly reduced.
The ease by which complex Excel models can be altered to accept uncertain inputs, and the ease with which the simulations can be used to determine the outputs’ sensitivity to input variation, make @RISK a valuable tool for pricing and investment strategy.
Randy Heffernan is vice president of Palisade Corporation, which specializes in risk and decision analysis software. Palisade’s most well-known product is @RISK, which is an add-in to Microsoft Excel and provides Monte Carlo simulation, optimization, and other risk analysis techniques.
The content & opinions in this article are the author’s and do not necessarily represent the views of AltEnergyMag
Comments (0)
This post does not have any comments. Be the first to leave a comment below.
Featured Product
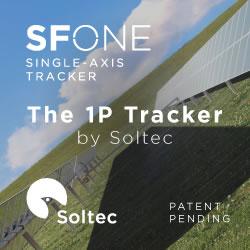