We think the satellite-image based Virtual Irradiance approach is a scalable and reliable solution to the problem of understanding solar PV performance.
How Virtual Irradiance Tops Relative Performance Analysis
Shawn Kerrigan | Locus Energy
One of the first steps in most analyses of solar PV systems is to determine expected system production based on the weather the system has experienced. One approach is to use the production from clusters of solar PV systems to estimate the weather adjustment, an approach involving relative performance algorithms. Locus Energy, a solar monitoring and data analytics platform provider for the residential, commercial and utility sectors, however, has evolved past using relative performance algorithms that compare the performance of a solar PV system with that of its neighbors, replacing this approach with a satellite imagery-based Virtual Irradiance system.
Locus Energy’s Virtual Irradiance (VI) satellite-based irradiance tool is one of three elements in its PVIQ Suite, which provides advanced performance analytics enabling asset managers to boost productivity, reduce operations and management costs and mitigate risk. Locus’ VI tool allows asset managers to easily identify which systems are not meeting performance expectations. The other PVIQ elements include an analysis tool to quantify system losses from specific effects like snow or shading, and an Excel plug-in that allows users to stream data directly into spreadsheets, dramatically reducing the time required to prepare performance reports.
VI uses Locus’ proprietary algorithms to provide highly accurate, ground-level irradiance data across an install base or geographic region. Because it measures the amount of sunlight, or “fuel,” that is striking a system at a particular time and place, VI is the first step in assessing system performance. We feel well-qualified to explain here why Locus Energy made the leap from relative performance algorithms to a satellite-imagery based Virtual Irradiance system, especially given that we were one of the early pioneers in developing relative performance algorithms and hold three patents in this area.
What’s The Problem With Relative Performance Algorithms?
As we refined our relative performance algorithms, we found there were several fundamental drawbacks that limited the analysis application. Below we’ll outline how the algorithms work and why they have inherent limitations.
The basic approach for all solar PV relative performance algorithms is as follows:
- For a given location, find all solar PV systems within a given distance
- Normalize the performance of the nearby systems by their expected output level
- Remove outliers or otherwise non-comparable systems (e.g., sites with clear production problems, data that looks incorrect, different technologies, etc.)
- Compare the normalized benchmark of selected sites
While the specifics of the algorithm can change (e.g., dynamic distance criteria, normalization approaches, outlier removal or system selection criteria, etc.), the basic idea of selecting nearby sites, normalizing, selecting a best-fit subset and then comparing to a baseline is core to relative performance algorithms. These types of algorithms suffer from four key drawbacks that limit their usefulness and accuracy for solar benchmarking and analysis:
- Limited coverage areas: The algorithm is limited to areas with a significant number of solar PV systems that pass all the filters. Even with 70,000 sites under monitoring (one of the largest data sets in the marketplace), Locus has found that having enough comparison sites can be a challenge. If we limit ourselves to areas with many solar PV sites to get a higher-quality result, we won’t be able to assess solar PV sites in less dense locations. If we allow less density, the algorithm error increases significantly. Moreover, there are often sites without any good comparison sites nearby.
- Nearby sites do not always experience similar weather: In some locations nearby sites experience very different weather due to microclimates (e.g., around San Francisco, or other coastal versus nearby inland site situations). Comparing across long distances is valid in some areas, and inaccurate in others. On the other hand, using a very short distance for comparison limits the comparable solar PV sites, reducing the number of locations for which results can be calculated and increasing the level of error.
- Many sources of error affect all sites in an area together: The goal of relative performance is to determine the weather based on solar PV system output, assuming the collective set of comparison sites represents weather effects and idiosyncratic performance issues wash out. Unfortunately, many factors that affect solar performance will affect all solar PV systems in an area together, such as snow or dust/soiling covering the panels. Soiling is particularly problematic because it affects all systems in an area fairly consistently and slowly over time, making it hard to detect and neutralize. These types of problems can cause relative performance algorithms to underestimate the actual sunlight that an area received.
- Different sites behave differently under the same weather conditions: Different solar PV systems will react differently to the same weather conditions. For example, if the weather is cloudy in the morning and clear in the afternoon, east-facing sites will be more negatively impacted than west-facing sites, skewing the benchmark comparison set. Thin-film sites will perform better on a relative basis than crystalline silicon sites under cloudy conditions, because they work relatively better with diffuse light. Thin-film and crystalline silicon also perform differently based on temperature. These types of problems can be partially addressed by restricting the criteria for comparison sites (e.g., same orientation and technology type), but that requires having all the necessary criteria metadata and further reduces the applicable data set, reducing the number of locations that can be analyzed and increasing error by shrinking the comparison set.
All of the above issues limit the locations where relative performance algorithms can be used to areas with a high density of similar systems, and even then the error from the output can be significant and confidence intervals on the output very wide.
As we dug deeper into this problem, we realized there had to be a better way.
Virtual Irradiance -- Accurate Unbiased Sunlight Data Anywhere
We realized that we needed an accurate, unbiased solution that could provide sunlight data wherever we were monitoring a solar PV site. Satellite imagery-based models can deliver on these objectives, so we set out to build a world-class satellite-based irradiance model. The idea behind the model is straightforward -- sunlight reaching the surface of the Earth is a function of:
- The sun’s position, which we can calculate with a bit of astronomy
- The impact of the atmosphere on incoming sunlight. This is primarily driven by cloud cover, which we can see and model based on satellite imagery.
Virtual Irradiance provides an answer to the question of how much sunlight reached a solar PV system that is more accurate and reliable than what is achievable with relative performance algorithms. Satellite-based models like Virtual irradiance are not without sources of error (see error discussion here), but they provide a large improvement over relative performance approaches.
We are about to release the sixth generation of our Virtual Irradiance model, and with each release we have made marked improvements to the system’s accuracy. We’re excited to announce that with our new Model 6 we’ve reached new heights, with a median monthly error of 2.6 percent and a 90th percentile monthly error of 5.7 percent. Accuracy is even higher in warm, sunny locations like California, which is where a lot of solar PV systems are installed. Notably, most commercial irradiance sensors have 5 percent baseline measurement inaccuracy, plus additional error from soiling, downtime and any miscalibration, so Virtual Irradiance performs similar to or better than many physical irradiance sensors in the field.
We think the satellite-image based Virtual Irradiance approach is a scalable and reliable solution to the problem of understanding solar PV performance, and we’re excited about the wide range of applications for this technology.
The content & opinions in this article are the author’s and do not necessarily represent the views of AltEnergyMag
Comments (0)
This post does not have any comments. Be the first to leave a comment below.
Featured Product
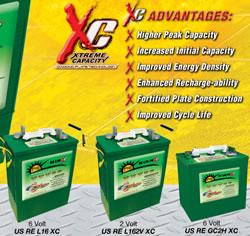